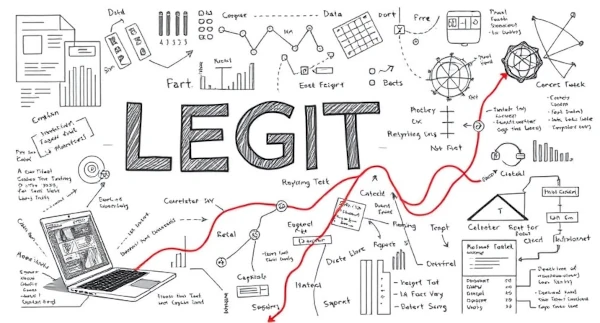
Artificial intelligence (AI) is everywhere—from chatbots and recommendation systems to autonomous vehicles. At the heart of these groundbreaking technologies lies data annotation, a critical process that powers AI systems. But as the demand for AI grows, so does the scrutiny surrounding data annotation technology. Is it reliable? Are the methods ethical? These are valid questions businesses and individuals are asking.
Let’s dive deep into data annotation tech, exploring its legitimacy, challenges, and benefits, so you can make informed decisions in this fast-evolving field.
What is Data Annotation Technology?
Data annotation technology involves labeling datasets so machines can understand and interpret information more effectively. It serves as the foundation for supervised learning, a core method in machine learning.
Types of Data Annotations
- Text Annotation: Adding tags to text data, such as identifying entities (e.g., names, dates) or sentiments.
- Image Annotation: Labeling objects in images, crucial for facial recognition and medical imaging.
- Audio Annotation: Tagging sounds, speech, and language for voice assistants.
- Video Annotation: Identifying actions or objects in videos, used in autonomous vehicles.
Applications
From medical imaging to retail analytics, data annotation technology fuels diverse industries, proving its indispensability in modern AI development.
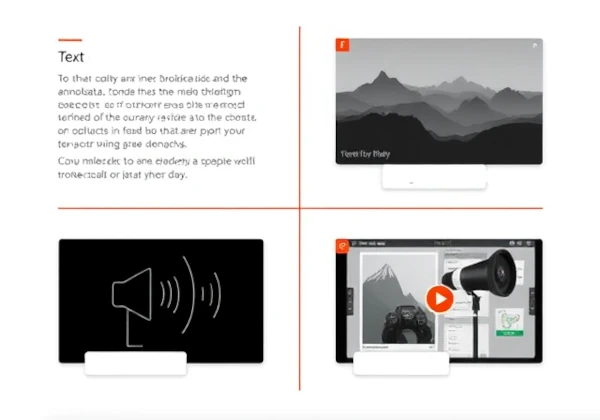
The Role of Data Annotation in AI Development
AI systems rely on annotated data to learn and predict outcomes. Without accurate annotations, these systems fail to deliver reliable results. For instance, chatbots like Siri and Alexa depend on text and audio annotations to process human language.
High-quality annotations ensure:
- Precise AI predictions
- Enhanced user experience
- Reduced errors in critical applications like healthcare or autonomous driving
The Controversy Around Data Annotation Tech
Despite its importance, data annotation faces skepticism due to concerns like:
- Ethical Sourcing: Are datasets obtained with proper consent?
- Inconsistent Accuracy: Low-quality annotations can derail AI performance.
- Exploitation of Workers: Reports of unfair labor practices among annotators raise questions about the industry's ethical standards.
How to Evaluate the Legitimacy of Data Annotation Tech
To determine the reliability of data annotation services:
- Check Certifications: ISO certifications and compliance with data regulations like GDPR indicate legitimacy.
- Review Vendor History: Established companies with a track record of successful projects are more trustworthy.
- Demand Transparency: Reliable vendors disclose their sourcing and labor practices.

The Impact of Poor Annotation Practices
Bad annotations lead to flawed AI systems. Imagine an autonomous vehicle misidentifying a pedestrian due to inaccurate video labeling—this could lead to fatal consequences. Consistently poor practices damage businesses, hinder AI advancements, and erode public trust.
Ethical Issues in Data Annotation
The industry grapples with ethical dilemmas:
- Labor Exploitation: Annotators, often in developing countries, sometimes face poor wages and working conditions.
- Data Privacy: Mishandled datasets can result in breaches or misuse of sensitive information.
Solutions
Organizations must adopt fair labor policies and invest in secure data management systems to mitigate these concerns.
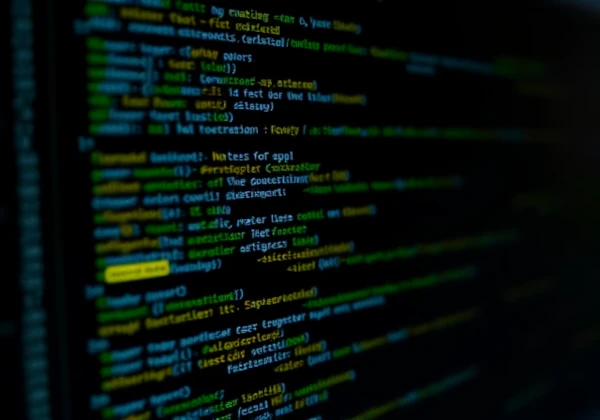
Legitimate Use Cases of Data Annotation Tech
Examples of legitimate and impactful uses include:
- Healthcare: Annotated medical images aid in early diagnosis.
- Autonomous Vehicles: Object recognition relies on meticulously labeled video data.
- Retail and E-commerce: Chatbots and product recommendations improve customer experiences.
How Companies Can Choose Reliable Data Annotation Services
Selecting a trustworthy vendor is crucial. Look for:
- Clear service agreements
- Demonstrated expertise in your industry
- Ethical sourcing practices
Avoid vendors with vague policies or inconsistent project outcomes.
Benefits of Legitimate Data Annotation Tech

Investing in reliable annotation services offers several advantages:
- Enhanced AI performance
- Faster project completion
- Better resource utilization
Future Trends in Data Annotation Technology
The industry is moving toward:
- Automation: AI-driven annotation tools to reduce manual effort.
- Hybrid Models: Combining automated tools with human oversight.
- Growth in Demand: With AI adoption increasing, the annotation market is set for exponential growth.
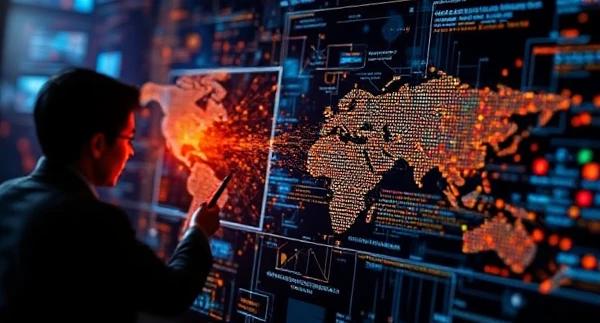
Conclusion
Data annotation technology is not only legitimate but vital for the success of AI and machine learning applications. However, businesses must prioritize ethical practices and high-quality services when choosing annotation providers. The right approach ensures AI systems are effective, accurate, and trustworthy.
FAQs
What is data annotation technology?
Data annotation technology involves labeling data like text, images, or videos to train machine learning and AI models. It ensures the data is structured and meaningful for accurate model predictions.
Why is data annotation essential for AI?
Data annotation is essential for AI because it provides labeled datasets needed to train models, enabling them to recognize patterns and make accurate predictions. Without annotation, AI systems cannot effectively interpret raw data.
How can I ensure the legitimacy of data annotation services?
To ensure legitimacy, verify the service provider's experience, client reviews, and data security practices. Additionally, request sample work to assess quality and compliance with your project requirements.
Are there ethical concerns in data annotation?
Yes, ethical concerns in data annotation include privacy violations, exploitation of annotators, and biases in labeled data. Ensuring transparency, fair labor practices, and unbiased datasets helps address these issues.
What are the future trends in data annotation technology?
Future trends in data annotation technology include increased use of AI-powered automation, real-time annotation, and improved tools for handling complex data types like 3D images and video. Emphasis on scalability and ethical practices will also grow.